Data Science in Finance: Predictive Modeling and Risk Management

Introduction
In the fast-paced world of finance, data science has emerged as a game-changer, revolutionizing traditional practices and unlocking new avenues for predictive modeling and risk management. By harnessing vast datasets and leveraging advanced analytical techniques, data scientists in the financial sector are equipped to extract valuable insights, forecast market trends, and mitigate risks with unprecedented accuracy.
The convergence of data science and finance has ushered in a new era of innovation, where predictive modeling algorithms and quantitative analysis techniques enable financial institutions to make informed decisions in real-time. From optimizing investment strategies to assessing credit risk and detecting fraudulent activities, the applications of data science in finance are diverse and far-reaching.
This article delves into the realm of "Data Science in Finance: Predictive Modeling and Risk Management," exploring the foundational principles, cutting-edge methodologies, and ethical considerations that define this dynamic field. As we navigate through the intricacies of financial data analytics, we uncover how data science is reshaping the landscape of finance, driving efficiency, and facilitating smarter decision-making processes.
Foundations of Data Science in Finance
In the realm of finance, data science serves as the cornerstone for unlocking actionable insights from complex datasets. At its core, data science encompasses a multidisciplinary approach that integrates statistics, machine learning, and computer science to analyze and interpret financial data. The foundational principles of data science in finance include:
Data Collection and Preprocessing:
Data collection is the initial step in the data science pipeline, where vast amounts of financial data are gathered from various sources such as market exchanges, financial institutions, and regulatory bodies. This raw data undergoes preprocessing, including cleaning, filtering, and normalization, to ensure its quality and consistency for analysis.
Exploratory Data Analysis (EDA):
Exploratory Data Analysis (EDA) involves visualizing and summarizing the characteristics of financial datasets to gain insights into underlying patterns and relationships. Techniques such as statistical summaries, data visualization, and correlation analysis help data scientists identify trends, anomalies, and potential areas for further investigation.
Feature Engineering:
Feature engineering is the process of selecting, transforming, and creating new features from raw data to improve the performance of predictive models. In finance, feature engineering techniques may involve extracting relevant financial indicators, creating lagged variables, and encoding categorical variables to capture meaningful information for modeling.
Predictive Modeling in Financial Markets
Predictive modeling lies at the heart of data science applications in financial markets, enabling analysts to forecast future outcomes based on historical data and market trends. Key aspects of predictive modeling in finance include:
Time Series Analysis:
Time series analysis is a fundamental technique used to model and forecast financial data that evolves over time. Methods such as autoregressive integrated moving average (ARIMA), exponential smoothing, and Prophet models are employed to analyze time-dependent patterns and make predictions for future market movements.
Machine Learning Algorithms:
Machine learning algorithms play a pivotal role in building predictive models for financial applications. Supervised learning algorithms, including regression, classification, and ensemble methods, are used to predict stock prices, identify trading signals, and classify credit risk. Additionally, unsupervised learning techniques such as clustering and anomaly detection assist in uncovering hidden patterns and anomalies in financial data.
Sentiment Analysis and Natural Language Processing (NLP):
Sentiment analysis and Natural Language Processing (NLP) techniques are leveraged to analyze textual data from financial news, social media, and analyst reports. By extracting sentiment and sentiment-related features from textual data, data scientists can gauge market sentiment, identify emerging trends, and assess the impact of news events on financial markets.
Predictive modeling empowers financial institutions to make data-driven decisions, optimize investment strategies, and manage risks more effectively. By leveraging advanced analytical techniques, data scientists can uncover valuable insights from financial data, providing a competitive edge in today's dynamic and rapidly evolving markets.
What are the Risk Management and Quantitative Analysis?
Risk management is a critical aspect of financial operations, and data science plays a pivotal role in quantifying and mitigating various forms of risk. Key components of risk management and quantitative analysis in finance include:
Portfolio Optimization:
Portfolio optimization aims to construct investment portfolios that maximize returns while minimizing risk. Data science techniques, such as mean-variance optimization and modern portfolio theory, help investors allocate assets effectively based on their risk tolerance and investment objectives. By analyzing historical returns and covariance matrices of asset classes, data scientists can identify optimal portfolio allocations that balance risk and return.
Value at Risk (VaR) Modeling:
Value at Risk (VaR) modeling is a quantitative technique used to estimate the maximum potential loss of a portfolio over a specified time horizon at a given confidence level. Data scientists employ statistical methods, such as parametric, historical simulation, and Monte Carlo simulation approaches, to calculate VaR for different asset classes and assess the portfolio's exposure to market risk.
Stress Testing:
Stress testing involves subjecting a financial system or portfolio to adverse scenarios to evaluate its resilience and vulnerability to extreme market conditions. Data science techniques facilitate the creation of stress test scenarios, simulating market shocks, economic downturns, and geopolitical events. By analyzing the impact of stress events on portfolios, financial institutions can identify potential weaknesses and implement risk mitigation strategies.
Applications in Investment Strategies
Data science is transforming the landscape of investment strategies, empowering investors to make informed decisions and generate alpha in financial markets. Key applications of data science in investment strategies include:
Quantitative Trading Strategies:
Quantitative trading strategies leverage data science techniques to develop algorithmic trading models that systematically exploit market inefficiencies and generate profits. Data scientists use machine learning algorithms to analyze market data, identify trading signals, and execute trades with minimal human intervention. High-frequency trading (HFT) strategies, arbitrage opportunities, and trend-following models are examples of quantitative trading strategies deployed in financial markets.
Factor Investing and Smart Beta Strategies:
Factor investing aims to capture specific risk premia or factors that drive asset returns, such as value, momentum, and quality. Data science facilitates the identification and construction of factor-based investment strategies using quantitative methods and statistical analysis. Smart beta strategies, which seek to outperform traditional market-capitalization-weighted indices, rely on data-driven insights to design rules-based portfolios that tilt towards factors with historically higher returns.
Alternative Data and Predictive Analytics:
Alternative data sources, including satellite imagery, social media sentiment, and web traffic data, are increasingly utilized in investment research to gain unique insights into companies, industries, and economic trends. Data scientists employ predictive analytics techniques, such as machine learning and natural language processing, to extract actionable signals from alternative data sources and generate alpha for investment portfolios.
The integration of data science techniques into risk management practices and investment strategies marks a paradigm shift in the financial industry. By harnessing the power of quantitative analysis and predictive modeling, financial institutions can navigate complex market dynamics, optimize investment decisions, and mitigate risks effectively, paving the way for a more efficient and resilient financial ecosystem.
Credit Risk Assessment and Loan Portfolio Management
Credit risk assessment and loan portfolio management are vital functions within the financial sector, and data science plays a crucial role in enhancing their effectiveness. Key aspects include:
Credit Scoring Models:
Data science enables the development of credit scoring models that assess the creditworthiness of borrowers and determine the likelihood of default. Machine learning algorithms, such as logistic regression, random forests, and gradient boosting machines, analyze borrower characteristics, credit history, and financial metrics to assign credit scores. These models help financial institutions make informed lending decisions and manage credit risk effectively.
Loan Default Prediction:
Predictive modeling techniques are employed to forecast the probability of loan default based on historical data and borrower attributes. By analyzing factors such as income, employment history, debt-to-income ratio, and credit utilization, data scientists can identify high-risk borrowers and implement risk mitigation strategies, such as adjusting interest rates or tightening lending criteria.
Fraud Detection and Compliance
Fraud detection and compliance are paramount in safeguarding the integrity of financial systems, and data science offers sophisticated tools to detect fraudulent activities and ensure regulatory compliance. Key components include:
Anomaly Detection:
Data science techniques, such as anomaly detection algorithms and pattern recognition, are utilized to identify unusual patterns and outliers indicative of fraudulent behavior. By analyzing transactional data, user behavior, and network activity, data scientists can detect anomalies in real-time and flag suspicious activities for further investigation.
Anti-Money Laundering (AML) and Know Your Customer (KYC) Compliance:
Data science is employed to automate AML and KYC compliance processes, streamlining customer due diligence and transaction monitoring procedures. Machine learning algorithms analyze customer data, transactional patterns, and risk indicators to detect potential money laundering activities and ensure regulatory compliance. By leveraging data science, financial institutions can enhance their ability to identify and mitigate financial crimes while minimizing false positives and operational inefficiencies.
Incorporating data science into credit risk assessment, loan portfolio management, fraud detection, and compliance processes enhances the efficiency and effectiveness of financial institutions. By leveraging advanced analytics and predictive modeling techniques, financial institutions can make informed decisions, mitigate risks, and ensure regulatory compliance, thereby safeguarding the stability and integrity of the financial system.
Conclusion
The integration of data science into the realm of finance has revolutionized traditional practices, enabling financial institutions to make data-driven decisions, mitigate risks, and enhance operational efficiency. From predictive modeling in investment strategies to credit risk assessment and fraud detection, data science techniques have become indispensable tools in navigating the complexities of financial markets. In the bustling city of Delhi, where the financial landscape is dynamic and evolving, enrolling in a data science course in Delhi, Noida, Goa, Guwahati, Chennai, etc. equips professionals with the skills and knowledge to thrive in this data-driven industry. By mastering the principles of data science and its applications in finance, individuals can unlock new opportunities, drive innovation, and contribute to the growth of the financial sector in Delhi and beyond.
What's Your Reaction?
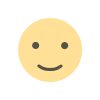



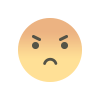

