Tackling the Biggest AI Challenges: Insights and Solutions
AI in engineering can apply to any part of the engineering spectrum - from generative AI to predictive analytics. Read more about AI in engineering.
Computer science and its various branches, such as machine learning, artificial intelligence, and deep learning, have become the main driving forces in several industries. As per the new report published by Goldman Sachs, on average, the firms invested about $76 billion in Artificial Intelligence in the current year, 2017, and this figure is likely to expand up to $200 billion by the end of the year 2025.
Artificial Intelligence (AI) is gradually being adopted in various sectors and is now impacting our lives and organizational practices. There is an explosive level of growth, but it comes with some problems. In this part, we will outline various issues in AI active today and how we could deal with these issues in the real world.
Leading Challenges in Artificial Intelligence
We will cover several issues, including some of the main faces of pursuing a career in AI and how we can tackle them.
1. Transparency and Explainability
Today, most artificial intelligence models, specifically deep learning algorithms, are usually called "black box." That is why their actions are hard for an outside observer to comprehend, which refers to decision-making. However, this is necessary to establish trust and be held accountable in the relationship.
Thus, to mitigate this issue of AI, one can focus on the subjects and research explainable artificial intelligence (XAI), which reveals information about model outputs. This will allow the users to comprehend the aspects the AI must consider when making its choices.
2. Mitigating Bias and Discrimination
This indicates that AI systems can become more prejudiced than societal norms if the style of the algorithm and data are prejudiced. This keeps out the idea of equity in using AI applications across human beings. Thus, the problem requires focused efforts toward developing non-biased algorithms and employing diverse training databases.
Businesses must work with other companies to acquire new datasets to ensure the model is trained on a diverse and accurate dataset. Furthermore, the issue is progressed by using bias detection and mitigation methods, which will contribute to ethical AI use.
3. Computing Power
The magnitude of power these hungry power algorithms consume is one thing that would keep most developers away. Artificial intelligence partly employs machine learning models and deep learning as its foundation, and they require numerous continuous cores and GPUs to function optimally.
4. Data Privacy and Security
It is critical to consider the main concerns of AI: the protection of data and privacy since AI involves the use of a vast amount of data for processing and learning. Measures call for ensuring data security, availability, and integrity to counter leakage, breach, and misuse. Over the past few years, the CCPA and GDPR have become popular legislation to regulate data protection, and any organization that intends to observe these rules must implement access permission control mechanisms, encryption, and audit ability.
Besides, applying differential privacy and federated learning approaches is mandatory to enhance the privacy of the algorithms and data. The fostering of trust by users in artificial intelligence and its systems is founded on proper and responsible handling of user data transparently and ethically.
5. Navigating Regulatory Challenges
AI poses new regulatory questions because of its speed of emergence and implementation. There is a saying that 'Governing with technology is governing technology.' This means that until the government demonstrates the right techniques in governance, AI technologies should also have the right administration to ensure that they are used safely. However, current governments usually react as AI applications are developed and implemented.
Coordinating the policy of these technologies' regulation and data handling is necessary due to the global nature of AI platforms. Governments need to help coordinate the development of private sectors through a system of regulation supporting innovations and preventing negative consequences and doubtful ethical standards when necessary.
6. Overcoming Technical Difficulties
The major common technical AI issues that organizations have to address during the implementation of AI include storage, security issues, and scalability. Due to the high volume of data, artificial intelligence professionals need to build robust structures that can accommodate this junction. In a way, the trust from the user's side will depend on the data safety and the protection of users' privacy across the AI life cycle.
An organization must adopt a scalable approach when it comes to the introduction of AI. They have to be ready to apply it to satisfy the rising business and customer demands. In practice, the use of advanced hardware technology like specifically designed AI chips and distributed computing systems for larger computing capability.
Conclusion
Solving the challenges of AI requires not only the identification of technical problems and their solutions but also the consideration of ethical issues. Of course AI provides a great deal of advantages and has virtually no limitations, but it also implies some concerns which we have to navigate. If we channel these concerns into efforts for effective, ethical, and open development of AI, we can make use of this technology in the pursuit of more efficient, innovative solutions for society.
What's Your Reaction?
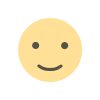



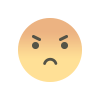

